Over the past few years, strides in material science, applied chemistry, manufacturing and logistics have caused energy storage system costs to drop significantly. However, as the industry matures, and “early adopters” give way to the “early majority”, the economic fine-tuning required to make storage projects as successful in the field as they seem on paper determines the fate of storage projects before they even leave the planning stage.
Energy storage has held the promise of solutions for companies that generate, transmit and distribute energy; this is especially true for anyone in the business of solar. Even end-users have come to see storage as a solution to issues such as balancing generation and consumption, reducing line losses, reviving grid assets after a blackout, avoiding expensive infrastructure upgrades, reducing curtailment of renewables and so on. Certainly, batteries can address these challenges, but most are inherently financial in nature and demand collaboration between the facilities, engineering and finance disciplines.
Enjoy 12 months of exclusive analysis
- Regular insight and analysis of the industry’s biggest developments
- In-depth interviews with the industry’s leading figures
- Annual digital subscription to the PV Tech Power journal
- Discounts on Solar Media’s portfolio of events, in-person and virtual
In California, for example, new solar projects are made possible through the addition of storage. However, if a developer recommends a storage system that’s too large or too small, the asset owner may consider the project a “failure” and that developer will not get the referral for the next project.
A grossly oversized storage system will do the job but never pay off or meet the internal rate of return (IRR) requirement. An undersized storage system will not do the job and suffer a similar economic fate. Software and analytics brought to bear early in the process provide ‘inoculation’ to storage miscalculation disease.
Expensive and time-consuming financial analysis (real fine-tuning) has relegated energy storage to the kind of mega-projects that could require a high level of analytical sophistication. In reality, this mostly manifests in cubicles full of analysts and shared drives filled with spreadsheets. The growth of the storage industry slows down when storage developers don’t have access to intelligent and easy-to-use tools with which to compare the possible value of a system against the costs of execution. Software and analytics are now driving a first-order change in this model, with machine learning and artificial intelligence quickly producing drastic second-order change.
The cost of analysis
Storage developers that are still in business do not just start pouring foundations and dispatching cranes on a whim. Every project opportunity is compared to a collection of possible project sizes and scenarios. This portfolio of project options is analysed to determine which ones will generate the most value, either by producing revenue or reducing cost. This process quickly becomes very complicated if you have to rely on spreadsheets or rudimentary online tools.
A quality analysis must comprehend load requirements, storage power output and storage capacity, system cost, the contribution of existing renewable resources, and the capability to scale. In effect, the more data a model can ingest and analyse, the higher the reliability of the analysis. Viable projects are compiled into a final report, which is presented to project stakeholders in a comprehensive format that helps decision-makers translate high-fidelity analysis with enough clarity to drive good management decisions.
This analytical process requires a large contingent of professionals tasked with data gathering, financial modelling, product research, and knowledge of available government incentives and their requirements. This exercise is labour-intensive, time-consuming and costly. Worse still, this human-led process is inherently vulnerable to error, which requires even more effort to correct – if discovered at all.
This analysis complexity and risk is what drives up the cost of prospective energy storage projects. It is no wonder that many projects never even make it to the concept stage, much less to construction and operation. Those that do are usually either from customers who are unconcerned with the real ROI of their systems, or big firms with the means to solicit large-scale opportunities and commensurate balance sheets to absorb the costs of identifying, vetting and proposing storage projects.
Machine learning: The great equaliser
Software and data analytics have radically transformed the dynamics of everything from video games to healthcare. The storage industry is now entering the fray. Within a few minutes, sophisticated algorithms can crunch a year’s worth of 15-minute energy consumption data, a region’s historical spot prices for wholesale power, the number of critical peak events promulgated by a grid operator during the previous summer, and the price-per-kilowatt of demand charges for a commercial electrical tariff into a tidy visual representation.
This still relatively simple example is not beyond the cognitive capacity of humans, it is just beyond the capability of humans to do quickly and accurately the first time. Advanced software and analytics are revolutionary because the commercial tools that do this work are available at prices even the sole proprietor of a business, storage or solar company can afford.
Individuals can now assess a portfolio of projects quickly and accurately and handle the entire process from project lead identification to analysis, and through to the proposal stage. As ‘the little guys’ leverage these tools to deliver projects at a lower price and faster, the market will become more dynamic.
The confluence of cheaper, more capable components and the democratisation of analytical prowess is the source of more capable systems, available for the same price, with less risk and more economic value. This potent cocktail is now propelling more energy storage projects beyond the proposal stage. But that is not where the story ends.
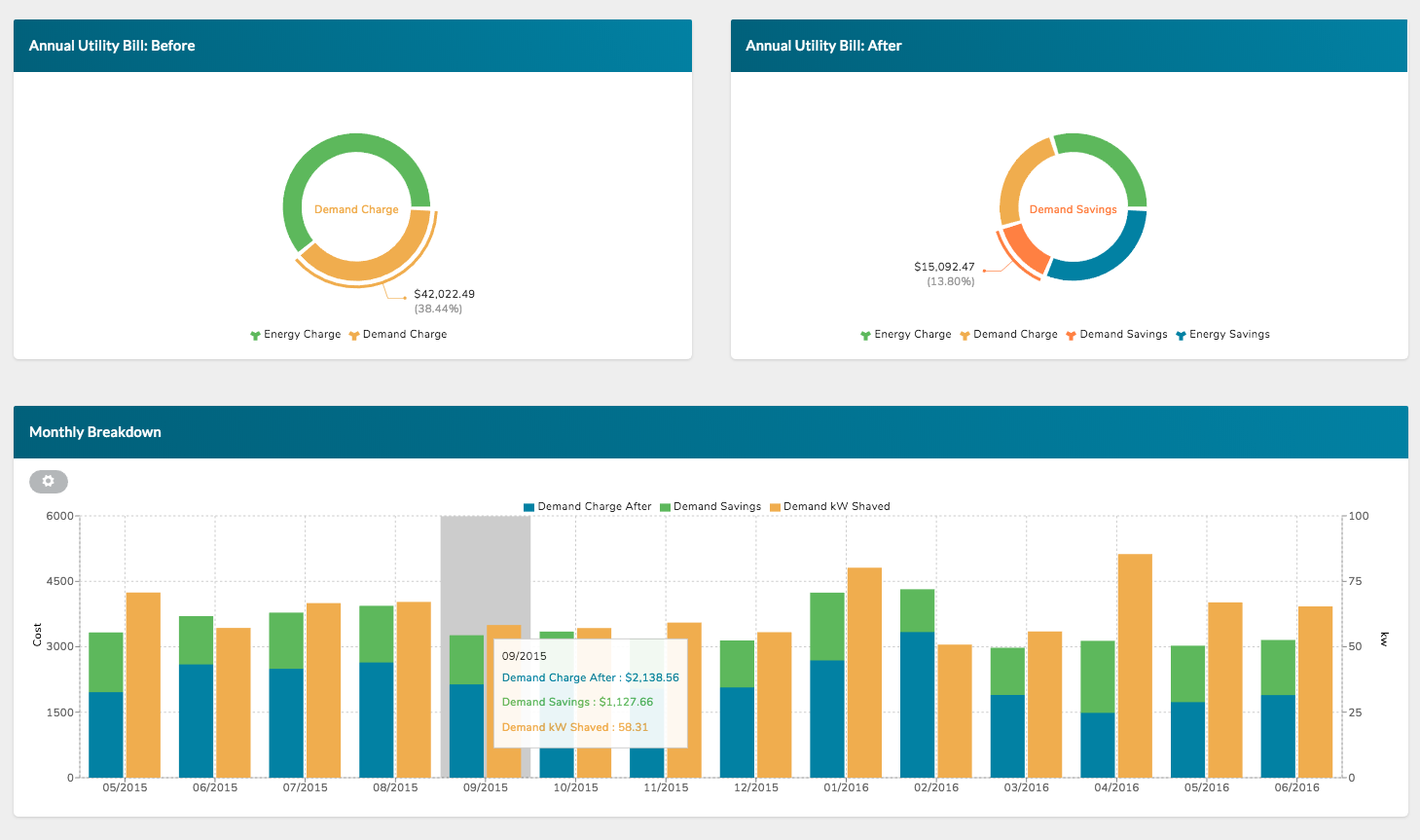
The value of computation in execution
Modern energy storage systems are capable of storing and discharging significant amounts of energy with high precision in fractions of seconds. However, the value of the asset can only be realised once these capabilities are engaged at the right time and the proper rate. Backup is a banner use case, but there is little computational complexity in waiting until an interruption in grid service is detected, isolating the local circuit from the grid, and discharging at the appropriate frequency until either the capacity of the system is exhausted or grid service returns. Backup is not trivial, but it is relatively simple.
Demand charge reduction, on the other hand, requires reliably predicting several data sets: when the next peak in electrical demand may occur, what other peaks could be expected during the day, how much solar production can be expected, and so on. Individually, these are complex computational models, but taken together and executed in real-time these models become as complex as they are valuable. To wit, additional complexity comes through the context of federal and state level incentives, such as the Investment Tax Credit (ITC) or Self Generation Incentive Programme (SGIP), offered across the United States and in California respectively. Solar-plus-storage projects claiming the ITC or SGIP incentives will forfeit the financial benefits of these programmes if the storage system is not charged exclusively by the associated, qualified renewable generation infrastructure.
Incurring demand charges will quickly and profoundly undermine (or even eliminate) an asset’s value proposition. Under electrical tariffs with a ratchet structure for demand charges, such a mistake can eliminate the expected savings of a demand charge reduction system for six months or more. As such, a storage system is dependent on solar generation, for example, to replenish during the charge cycle or intelligently and quickly adjust its peak shaving strategy. Storage management systems must ingest and comprehend real-time data sources such as weather forecasts, irradiance sensor readings, and on-site submeter outputs to achieve the necessary prediction accuracy required to deliver demand charge savings. Only by pushing this sophistication and insight to the storage devices themselves can modern machine learning methods reliably provide demand charge reduction.
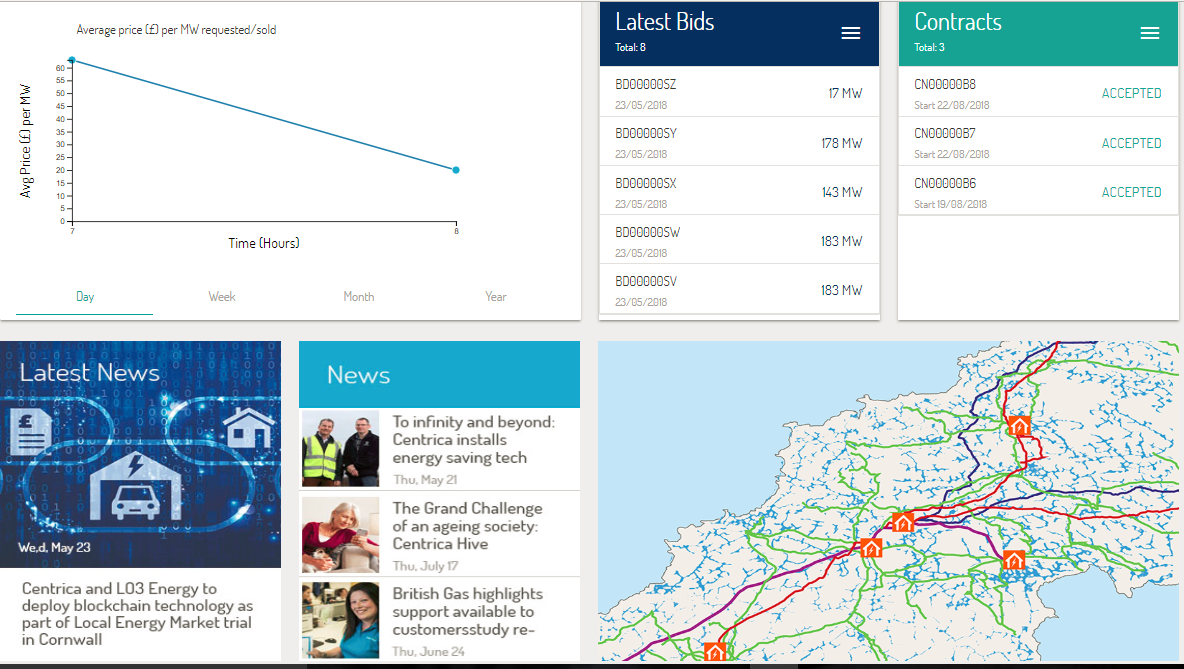
The importance of constants
Understanding whether a storage system is appropriately sized and capable of performing a given set of functions is critical in the planning process. Moreover, ensuring that a storage system has access to the fabric of data required to respond in real-time is crucial to the value-added operation of the system.
In all but the fewest cases, however, do the same people work on the former and the latter. Rarer still is the application of the same computational model in planning and operation. This discontinuity is one of if not the most significant contributors to stalling the storage market today.
To unleash the next level of value in the storage market, the assumptions used during the planning phase must be the same as the assumptions used in the finished system. Put another way, the realities of the working life of a system must serve as assumptions during the planning phase. It sounds obvious. Perhaps it sounds flippant. However, this bit of common sense is the exception, not the rule, in the storage industry today.
Time to stop talking about value-stacking
As an industry, we’ve had our fill of looking at and presenting value-stacking slides. Layering multiple applications is orders of magnitude more complex than what we’ve discussed above, and few initiatives ever make it past PowerPoint. Real world value-stacking demands that the cumulative advances in software and analytics perform at their limits, but only a handful of companies specialise in software and analytics to the extent required to know the limits, much less push them. Value-stacking, therefore, must go beyond executing on a given use case for a solar-plus-storage project and to co-optimise maximal operation with other often competing means of exploiting the asset.
An energy storage system may be intended to generate value through a combination of demand charge reduction, PV load-shifting, a demand response programme, and wholesale power market participation as a component of a virtual power plant (VPP). The software controlling the system must employ advanced analytics to anticipate possible savings or revenue across these four strategies continually, and determine in real-time which use case should be pursued with the available capacity.
For example, awareness of the utility tariff structure and historical performance of the system can be used to determine that there will not be an opportunity to peak shave the facility’s electrical demand on a given day. Instead, the system may opt to store the solar generation from the PV array and discharge it later in the day where the spread between the respective prices for power, accounting for system losses, would lead to an increase in the value of the solar energy produced.
The software controlling an energy storage system must continually leverage a variety of data sources as well as communicate with demand response servers, or distributed energy resource management systems (DERMS), to determine the likelihood of being able to participate in applications that may be more lucrative for the asset owner than merely shifting the PV production to a more valuable time-of-use period. If the system is too optimistic or aggressive about pursuing these opportunities, it may miss the chance to generate value by executing an alternative application. Storage software, therefore, must take into account a multitude of possible value streams, comprehend the likelihood of capturing them, enumerate their relative values and account for the consequences of pursuing any of them given the impact on equipment lifespan. This software now exists and will relieve executives and managers of their PowerPoint duties.
Extracting value
What got us here won’t get us there. The first generation of storage was built on chemistry, charge controllers and amp-hours. The next generation of storage is being built on massive heterogeneous datasets, machine learning and sensor networks. These new domains of expertise will not replace the old ones; instead, they will augment them and serve to unleash vast amounts of new value. In years past, we knew there was more value in storage. We could describe this value on paper (or in presentations). Today we have the technology to extract this value, and that makes this an exciting time for the entire energy storage value chain.
This piece was co-authored by Bryce Evans, head of customer and partner success for Pason Power. Prior to his current position, Bryce held several roles at Pason Power’s parent company, Pason Systems, contributing to software products providing data transport, hosting, and delivery for Pason’s energy clients.
This article originally appeared in PV Tech Power, the quarterly downstream solar technology journal from the makers of PV Tech and Energy-Storage.news. Subscribe to and download every issue of PV Tech Power, featuring ESN’s ‘Storage & Smart Power’ section dedicated to technology and market advancements that go beyond generation and deployment. Free of charge – registration details are required.
You can also download this article as a PDF to keep, along with other great feature articles, white papers and more, from the ‘Resources’ section of this site.